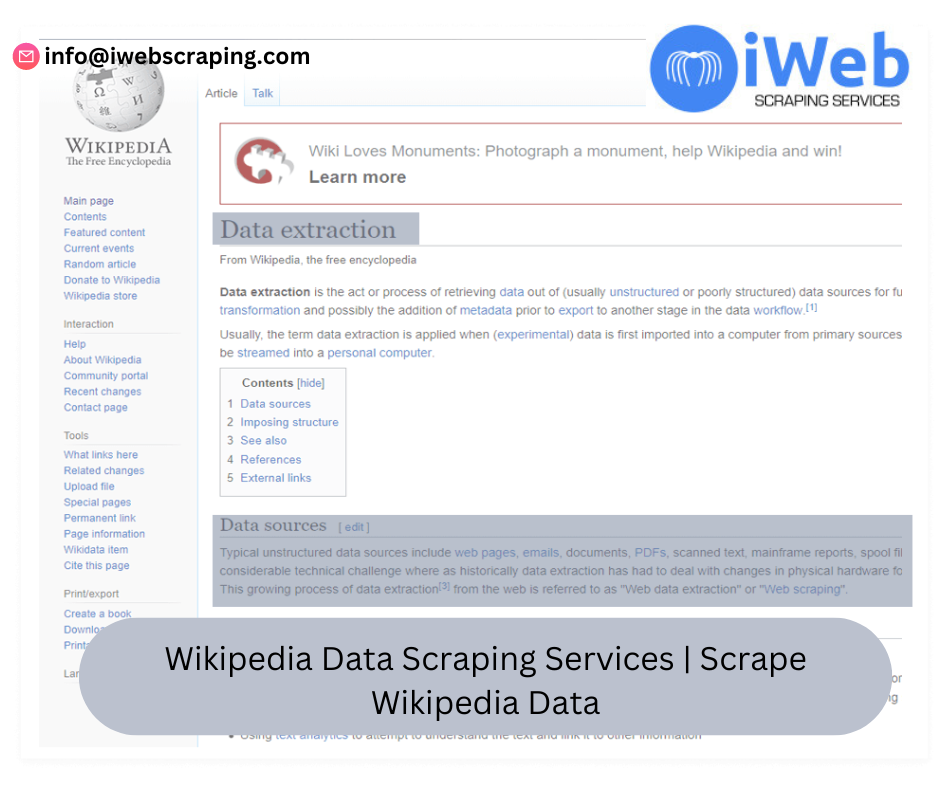
In the digital age, data is the lifeblood of decision-making. Businesses, researchers, and enthusiasts constantly seek reliable sources of information to fuel their insights. Wikipedia, with its vast repository of knowledge, serves as a goldmine for this purpose. However, manually extracting data from Wikipedia can be a daunting and time-consuming task. This is where Wikipedia data scraping services come into play, offering a streamlined and efficient way to harness the wealth of information available on the platform.
What is Wikipedia Data Scraping?
Wikipedia data scraping involves using automated tools and techniques to extract information from Wikipedia pages. This process bypasses the need for manual copying and pasting, allowing for the efficient collection of large datasets. Scraping can include extracting text, infoboxes, references, categories, and even multimedia content. The scraped data can then be used for various purposes, such as research, analysis, and integration into other applications.
Why Scrape Wikipedia Data?
Extensive Knowledge Base: Wikipedia hosts millions of articles on a wide range of topics, making it an invaluable resource for information.
Regular Updates: Wikipedia is continuously updated by contributors worldwide, ensuring that the information is current and reliable.
Structured Data: Many Wikipedia pages contain structured data in the form of infoboxes and tables, which can be particularly useful for data analysis.
Open Access: Wikipedia's content is freely accessible, making it a cost-effective source of data for various applications.
Applications of Wikipedia Data Scraping
Academic Research: Researchers can use scraped Wikipedia data to support their studies, gather historical data, or analyze trends over time.
Business Intelligence: Companies can leverage Wikipedia data to gain insights into market trends, competitors, and industry developments.
Machine Learning: Wikipedia's vast dataset can be used to train machine learning models, improve natural language processing algorithms, and develop AI applications.
Content Creation: Writers and content creators can use Wikipedia data to enrich their articles, blogs, and other forms of content.
How Wikipedia Data Scraping Works
Wikipedia data scraping involves several steps:
Identify the Target Pages: Determine which Wikipedia pages or categories contain the data you need.
Select a Scraping Tool: Choose a suitable web scraping tool or service. Popular options include Python libraries like BeautifulSoup and Scrapy, as well as online scraping services.
Develop the Scraping Script: Write a script that navigates to the target pages, extracts the desired data, and stores it in a structured format (e.g., CSV, JSON).
Handle Potential Challenges: Address challenges such as rate limiting, CAPTCHA verification, and dynamic content loading.
Data Cleaning and Processing: Clean and process the scraped data to ensure it is accurate and usable.
Ethical Considerations and Legal Compliance
While Wikipedia data scraping can be incredibly useful, it is essential to approach it ethically and legally. Here are some guidelines to follow:
Respect Wikipedia’s Terms of Service: Ensure that your scraping activities comply with Wikipedia’s terms of use and guidelines.
Avoid Overloading Servers: Implement rate limiting to prevent overwhelming Wikipedia’s servers with too many requests in a short period.
Credit the Source: Always credit Wikipedia as the source of the data and provide links to the original pages where possible.
Privacy Concerns: Be mindful of any personal information that might be present in the scraped data and handle it responsibly.
Choosing the Right Wikipedia Data Scraping Service
Several factors should be considered when selecting a Wikipedia data scraping service:
Reputation: Choose a service with a proven track record and positive reviews from users.
Customization: Look for services that offer customizable scraping solutions tailored to your specific needs.
Data Quality: Ensure the service provides clean, accurate, and well-structured data.
Support and Maintenance: Opt for services that offer ongoing support and maintenance to address any issues that may arise.
Conclusion
Wikipedia data scraping services open up a world of possibilities for accessing and utilizing the vast amounts of information available on the platform. Whether for academic research, business intelligence, machine learning, or content creation, these services provide a powerful tool for extracting valuable insights. By adhering to ethical practices and legal guidelines, users can harness the full potential of Wikipedia data to drive innovation and informed decision-making.
Write a comment ...